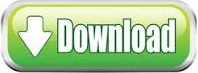

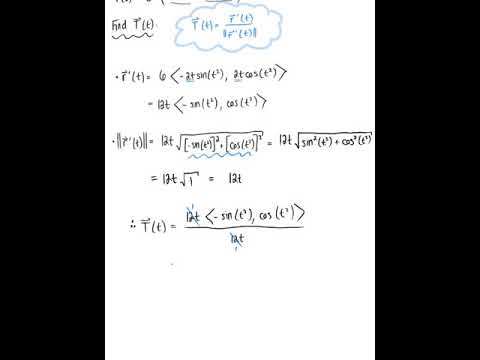
In cancer, due to deposition of aligned extracellular matrix proteins solid, stiff tumours can be recognized on the macroscale by palpation and similar techniques 7. Mechanical forces are important for cell migration, interaction of cells with their environment, tissue morphogenesis and in various forms of disease 1, 2, 3, 4, 5, 6. In addition, the maps enabled investigation of the relationship of the input variables. Our approach was able to distinguish between estrogen treated, control and resveratrol treated cells in an unsupervised manner. These data were then used as input for SOMs. We show changes in mechanical properties due to treatments, as estrogen softened the cells, while resveratrol led to an increase in cell stiffness and viscosity. We propose the use of self-organizing maps (SOMs) as unsupervised artificial neural network applied to mechanical measurements performed via AFM on epithelial breast cancer cells treated with different substances that affect estrogen receptor signalling. Together with the need to perform many measurements for statistical significance and to probe wide enough areas in tissue structures, the application of machine learning and artificial neural network techniques to automatically classify AFM datasets has received interest recently. These measurements often need skilful users, physical modelling of mechanical properties and expertise in data interpretation. Atomic Force Microscopy (AFM) is a widely utilized tool to study cell mechanics. Cancer cells show altered mechanical phenotypes compared to their healthy counterparts.
Principal unit normal vector 2d free#
Cell mechanical properties have been proposed as label free markers for diagnostic purposes in diseases such as cancer.
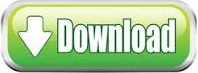